Navigating AI Patent Protection: Strategic Considerations for Biotech Companies
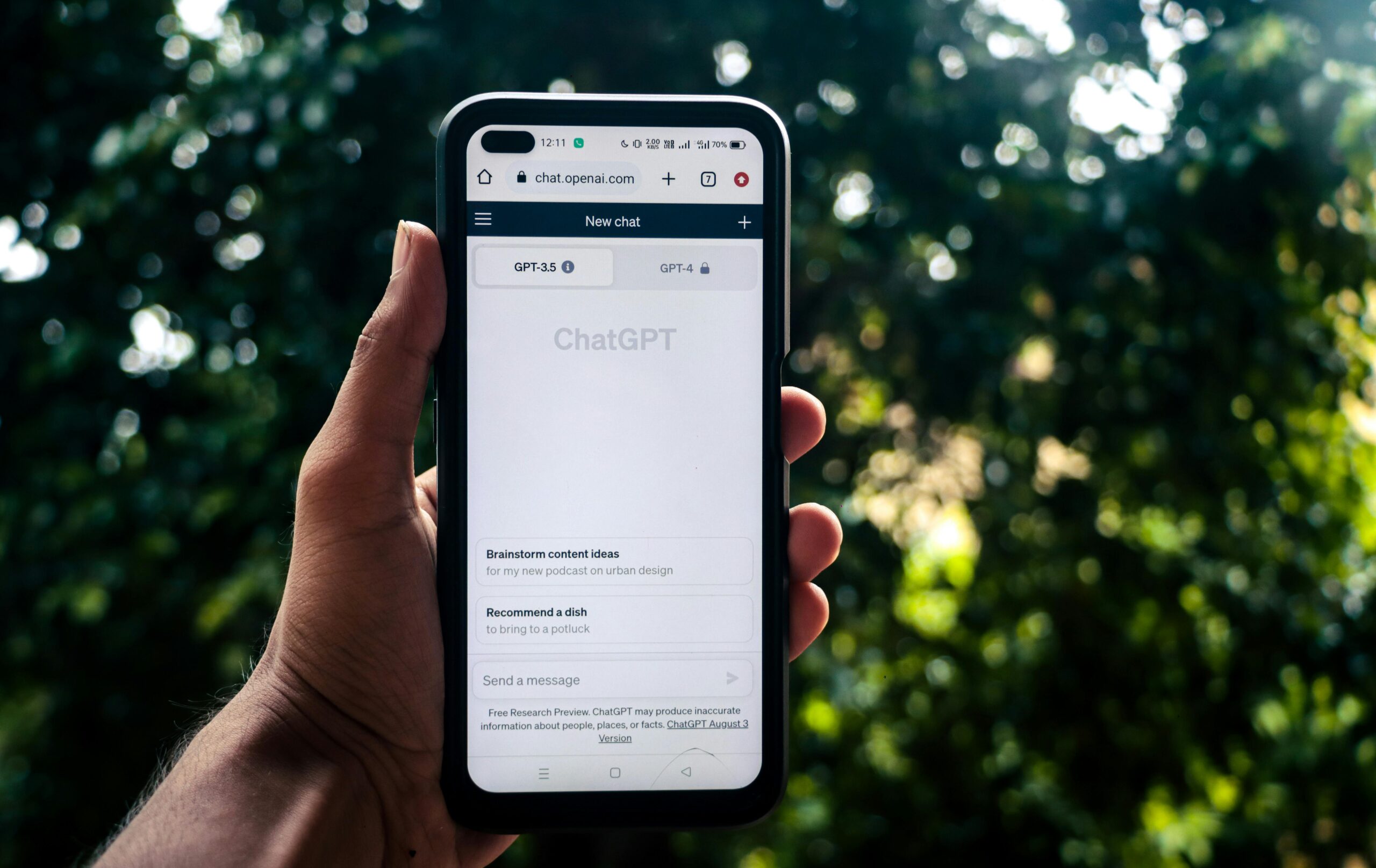
As artificial intelligence (AI) continues transforming the biotechnology landscape, protecting AI-related innovations has become increasingly critical for companies in this space. However, securing patent protection for AI technologies presents unique challenges that require careful consideration and strategic approaches. This article provides practical guidance for effectively assessing and protecting AI innovations while considering the legal framework in three major jurisdictions, including the United States, Europe, and China.
Patentable Applications of AI in Biotechnology
Before diving into specific jurisdictional requirements, it’s helpful to understand the types of AI innovations that may be patentable in the biotechnology space. These generally fall into several categories:
1. Drug Discovery and Development
- AI systems that predict drug-target interactions with improved accuracy
- Novel machine-learning architectures optimized for molecular property prediction
- AI-driven methods for identifying new drug candidates from existing compound libraries
- Systems that predict drug side effects or toxicity based on molecular structure
- Methods for optimizing drug formulations using machine learning
2. Protein Analysis and Engineering
- AI models that predict protein structure from sequence data with specific technical improvements
- Systems for predicting protein to protein interactions using novel neural network architectures
- Methods for designing proteins with desired properties using machine learning
- AI-driven approaches to antibody design and optimization
- Systems that predict protein stability or folding pathways
3. Diagnostic and Medical Imaging
- AI systems that enhance medical image quality or reduce processing time
- Novel neural network architectures for detecting specific biological markers
- Methods for integrating multiple data types to improve diagnostic accuracy
- Systems that automate the analysis of pathology images with specific technical implementations
- AI approaches that reduce false positives and/or negatives in medical screening
4. Genomic Analysis
- AI systems that identify genetic variants with improved accuracy or efficiency
- Novel methods for predicting gene function or regulation
- Systems that integrate multiple genomic data types in technically innovative ways
- Methods for predicting phenotype from genotype using specific machine learning approaches
- AI-driven approaches to analyzing gene expression data
5. Laboratory Automation and Optimization
- AI systems that optimize experimental conditions in real time
- Methods for automating complex laboratory workflows with specific technical improvements
- Systems that predict experiment outcomes to reduce failed trials
- AI-driven quality control systems with novel technical implementations
- Methods for optimizing bioprocess parameters using machine learning
6. Clinical Trial Design and Analysis
- AI systems that optimize patient selection using multiple data sources
- Novel methods for predicting trial outcomes or identifying potential issues
- Systems that analyze trial data in real time with specific technical implementations
- Methods for reducing bias in trial design using machine learning
- AI approaches to monitoring patient responses with improved accuracy
In each of these categories, the key to patentability often lies in the technical details of how the AI system achieves its results rather than the biological application alone. For example, while a basic application of a standard neural network to analyze protein sequences might not be patentable, a novel network architecture that addresses the challenges of protein sequence analysis could be. Similarly, while using AI to predict drug properties alone may not be patentable, a specific technical implementation that improves prediction accuracy through innovative data processing or model architecture might be.
Understanding the United States Patent and Trademark Office Approach
The United States Patent and Trademark Office (USPTO) evaluates AI patent applications through the lens of the established subject matter eligibility framework under 35 U.S.C. § 101, as refined by Supreme Court precedent and USPTO guidance. Understanding this framework is crucial for biotech companies as it provides specific pathways for protecting AI innovations while navigating potential eligibility challenges.
The USPTO’s analysis begins with whether the claimed invention falls within one of the four statutory categories: process, machine, manufacture, or composition of matter. This first hurdle for AI innovations in biotechnology is typically straightforward, as most implementations can be characterized as either processes or machines. However, the critical challenge emerges in the second phase of analysis, which examines whether the invention is directed to a judicial exception – specifically, abstract ideas in the case of most AI technologies.
The USPTO has identified specific groupings of abstract ideas that commonly arise in AI patents: mathematical concepts, mental processes, and particular methods of organizing human activity. Mathematical concepts are particularly relevant for AI patents, as they encompass mathematical relationships, formulas, equations, and calculations that often form the core of machine learning algorithms. However, the presence of mathematical concepts does not automatically render an invention ineligible. Instead, the USPTO employs a two-prong approach at Step 2A of the analysis.
Under Prong One, examiners determine whether the claims recite an abstract idea. For AI innovations in biotechnology, this often involves carefully considering whether the claimed elements could be characterized as mathematical calculations or mental processes. The key at this stage is to understand that while the underlying AI algorithms may be based on mathematical concepts, claims that do not explicitly recite mathematical relationships or calculations may avoid categorization as abstract ideas.
Prong Two provides the critical pathway for establishing patent eligibility by demonstrating that abstract ideas are integrated into a practical application. This integration can be shown through various means relevant to biotech applications, such as improvements to computer functionality, advances in specific technical fields like drug discovery or protein folding prediction, or implementation with specialized biological data processing hardware.
While there is a further step to the 101 framework, Step 2B, the practical reality is that details sufficient to establish a practical application at Step 2A, Prong Two, should be sufficient to navigate the 101 framework successfully. For example, in Ex Parte Hunnun, the Board found the claims eligible, because they detailed specific technical implementations, including a well-optimized recurrent neural network training system capable of using multiple GPUs and novel data synthesis techniques for efficient training data generation. In reversing the Examiner’s rejection, the Board indicated the analysis should have stopped at Prong 2. However, even when evaluated under Step 2B’s “significantly more” framework, the claims were also directed to patentable subject matter for similar reasons to the analysis under Step 2A, Prong 2.
The European Patent Office Framework
The European Patent Office (EPO) approaches AI patentability through a sophisticated framework that requires careful attention to both technical character and inventive step. Under Article 52(2) EPC, while mathematical methods and computer programs are excluded from patentability “as such,” AI innovations can establish patentability by demonstrating meaningful technical character through one of two fundamental pathways.
The first pathway involves technical application, often referred to as “Applied AI.” The AI method must transcend abstract mathematical operations to serve a specific, concrete technical purpose. The EPO has developed clear guidance on what constitutes a technical purpose, recognizing applications such as digital signal enhancement, speech recognition, efficient data encoding, and improvements to data structures that materially enhance computer functionality. In the biotech context, AI applications must do more than process biological data – they must demonstrate a direct technical impact on biological or medical processes.
The second pathway focuses on technical implementation, known as “Hardware AI.” This approach requires showing that the implementation choices are driven by the technical realities of computer architecture rather than purely mathematical considerations. Success in this pathway often involves demonstrating how the AI system has been specifically adapted to overcome hardware limitations or improve computational efficiency through novel technical means.
The EPO’s assessment of inventive step in AI cases follows what is referred to as the COMVIK approach for mixed-type inventions. This methodology begins by carefully distinguishing between technical and non-technical features in the claims. Non-technical features are further divided into those that contribute to producing a technical effect in the context of the invention and those that do not. This distinction is crucial because only technical features and contributing non-technical features can support an inventive step.
In practical terms, the COMVIK analysis unfolds in several stages. First, the examiner identifies the closest prior art based on the technical and contributing features. Then, the differences between the claimed invention and this prior art are analyzed to determine whether they make a technical contribution. Features that make no technical contribution, even if novel, cannot establish an inventive step under EPO practice.
The assessment of plausibility plays a significant role in AI patent applications at the EPO. The application must make it at least plausible that the claimed technical effect is achieved across the entire scope of protection sought. This requirement can be particularly challenging for AI inventions in the biotech sector, where the relationship between algorithmic processes and biological outcomes must be clearly established. While post-published evidence can sometimes support plausibility, the application must provide a credible technical foundation.
The China National Intellectual Property Administration (CNIPA) Framework
As artificial intelligence continues to reshape every corner of the biotechnology landscape, obtaining patent protection for AI-driven biotech inventions in China has taken on growing importance. Under the country’s Patent Law and the guidelines issued by CNIPA, these innovations are usually assessed as computer-implemented inventions. That classification means that each application still has to satisfy the familiar requirements of novelty, inventive step, and practical applicability while demonstrating a clear technical character. Merely invoking “AI” or “machine learning” will not suffice; the invention must show how and why it addresses a concrete problem in a technical manner.
In practice, CNIPA examiners pay particular attention to how an AI system is trained, the nature and format of the data it uses, and the system’s tangible benefits. This approach places a premium on detailed disclosure. Applicants are expected to explain, for instance, whether the AI model is processing genomic sequences, imaging data, or protein structures, and to specify how the data was collected, cleaned, and formatted. The training process itself must be described so that a person skilled in the art can understand how parameters were chosen, what algorithmic steps were followed, and how the system ultimately achieves its stated improvements—be those greater accuracy in disease diagnosis, faster screening of drug candidates, or more reliable protein-structure predictions.
Although AI-based biotech inventions are not formally treated as “unpredictable arts” in the same way that pharmaceuticals or complex gene therapies might be, the examiners often encourage applicants to include data that demonstrates the invention’s effectiveness. While such experimental results or comparative benchmarks are not strictly mandatory, they can help establish that the innovation meaningfully goes beyond conventional machine-learning approaches. At the same time, if human genetic or patient data is part of the invention, applicants must ensure compliance with China’s regulations on human genetic resources and personal information protection. This means they must balance sufficient disclosure to satisfy patent requirements with the obligation to protect privacy and adhere to administrative approvals for sensitive data.
Ultimately, CNIPA’s standards underscore the need for clarity and specificity. The claim language must capture the interplay between the AI method and the biological application in a way that spells out a true technical solution—rather than a mere abstraction or algorithmic exercise. When done successfully, AI-biotech patents can secure significant protections in China’s rapidly evolving life sciences sphere, so long as applicants articulate precisely what makes their invention both novel and technically advanced and substantiate those claims with robust disclosures.
Best Practices for Technical Disclosure
When preparing AI patent applications for global protection, careful attention must be paid to the distinct requirements of each jurisdiction. The description must address the USPTO’s practical application requirements under Section 101, the EPO’s technical character standards, and CNIPA’s detailed specifications for data, models, and training methods.
When describing the integration of AI systems with existing biotech workflows, the USPTO, EPO, and CNIPA practices demand particular attention to the implementation details. The description should clearly show how technical considerations drive the system’s architecture and implementation choices. This may include explaining how the AI system interfaces with laboratory information management systems (LIMS), detailing specific hardware configurations and adaptations, and demonstrating how technical constraints influenced the design decisions.
The description must establish clear causal links between AI processing steps and technical outcomes, moving beyond mere data processing to show how the system achieves concrete technical effects. For instance, in a drug discovery application, this might involve explaining how the AI system’s architecture was specifically designed to handle the unique characteristics of molecular data and how its output directly enables more efficient screening processes. The narrative should emphasize how each technical choice contributes to solving specific technical problems, whether in data processing efficiency, accuracy of predictions, or integration with existing laboratory workflows.
Sufficiency of disclosure is particularly scrutinized in AI cases at each patent office. While the requirement can generally be met by providing at least one way for a skilled person to carry out the invention, AI applications must be careful to establish clear causal connections between inputs and outputs. The description could include enough detail about model architecture, training requirements, and implementation considerations to make it plausible that the claimed technical effects can be achieved without undue experimentation.
Strategic Considerations: Patents vs. Trade Secrets
The detailed disclosure requirements for AI patents across jurisdictions create an important strategic tension for biotech companies. While robust patent protection requires comprehensive technical disclosure, including specific details about data characteristics, model architectures, and training methodologies, these elements often represent core competitive advantages that companies might prefer to maintain as trade secrets.
This tension is particularly acute in the biotech AI space for several reasons. First, the increased disclosure requirements mean that patent offices increasingly require experimental data and specific technical details that might otherwise remain confidential. Second, the rapid evolution of AI technology means that by the time a patent issues, the specifically disclosed implementation might be obsolete, while a trade secret could cover continuously updated versions. Third, the difficulty in detecting AI patent infringement might make trade secret protection more practical in some cases.
For biotech companies, additional industry-specific factors complicate this decision:
1. Training Data Considerations
- Proprietary datasets of protein-ligand interactions or clinical trial results often have significant competitive value
- Custom data preprocessing methods for biological data might be more valuable as trade secrets
- The way genetic or molecular data is formatted and normalized can be critical IP
- Historical patient data and biomarker correlations might be better protected as trade secrets
2. Regulatory and Validation Requirements
- The need to validate AI systems for regulatory approval might require public disclosure anyway
- FDA submissions might provide competitors insight into trade secrets
- The requirement for explainable AI in clinical applications might necessitate public disclosure
- Quality control processes for biological data might need to be transparent
3. Platform Technology Considerations
- Core AI architectures optimized for biological data might be more valuable patented
- Specific implementations of biomarker detection algorithms might be better as trade secrets
- Methods for integrating multiple biological data types might warrant patent protection
- Techniques for handling sparse or noisy biological data might be key trade secrets
4. Commercial and Collaborative Factors
- The need to collaborate with pharmaceutical partners might favor patent protection
- Academic collaborations might require public disclosure of methods
- The ability to license the technology to multiple partners might favor patents
- Integration with existing biotech workflows might require sharing implementation details
Companies must weigh multiple strategic considerations when evaluating whether to seek patent protection or maintain trade secrecy. The ability to detect and enforce rights plays a crucial role – companies should consider whether infringement of their AI implementation could be detected from publicly available information and whether the detailed technical disclosure required for patent protection might reveal more to competitors than necessary. The relative difficulty of proving trade secret misappropriation versus patent infringement also can influence this decision.
The commercial lifespan of the innovation presents another key consideration. Companies must assess whether their specific technical implementation will remain valuable beyond a typical patent term, particularly given the rapid evolution of AI technology. In some cases, an innovation might be better maintained as a trade secret if it could provide a competitive advantage for longer than the 20-year patent term.
The competitive landscape significantly impacts this strategic choice. Companies should evaluate how likely competitors are to develop similar solutions independently – if independent development is likely, patent protection might be more valuable than trade secrecy. Additionally, they should consider whether patent protection would effectively block competitors from key technological space and whether disclosure through patenting might benefit the company by advancing the field in helpful directions.
Business strategy considerations also play a vital role. Companies must evaluate whether the technology represents a core competitive advantage or a more peripheral innovation. Patent protection might be particularly valuable for enhancing company valuation during investment or acquisition discussions and opening licensing revenue possibilities. The choice between patents and trade secrets should align with the company’s broader business objectives and commercialization strategy.
Many biotech companies discover that the most effective intellectual property strategy combines patent protection and trade secrets, particularly when dealing with AI innovations involving sensitive data. This hybrid approach recognizes that different aspects of an AI system deserve different forms of protection, with the choice often driven by both strategic considerations and regulatory requirements.
The complexity of this decision becomes particularly apparent when dealing with patient data and other regulated information. While a company might seek patent protection for its novel neural network architecture designed for analyzing medical images or predicting drug responses, the underlying training data often involves patient information protected by regulations like HIPAA in the United States or GDPR in Europe. These privacy regulations create natural boundaries between what can be publicly disclosed in a patent and what must remain confidential.
Patent applications involving AI systems trained on patient data require careful crafting to satisfy both disclosure requirements and privacy regulations. Companies must often find creative ways to demonstrate their technology’s effectiveness without revealing protected information. This might involve describing the technical implementation using anonymized examples, focusing on data structures and processing methods rather than specific training data, or providing validation results in ways that maintain individual privacy while still proving the invention’s utility.
The interplay between privacy regulations and disclosure requirements adds another dimension to the traditional patent versus trade secret analysis. Often, the aspects of an AI system that must remain confidential for regulatory compliance – such as detailed training datasets or specific patient-derived features – also provide significant competitive advantage. This alignment can make trade secret protection a natural choice for these components, while patent protection better serves the novel technical implementations that transform this sensitive data into valuable insights.
This sophisticated approach allows companies to build robust intellectual property portfolios that protect their innovations and ensure regulatory compliance. The patent components can establish broad protection for fundamental technical advances and facilitate licensing opportunities, while trade secrets preserve competitive advantages in implementation details and help maintain privacy compliance. As AI technology evolves and regulatory requirements become more complex, this nuanced strategy becomes increasingly valuable for biotech companies navigating the intersection of innovation, privacy, and intellectual property protection.
Conclusion
Successfully protecting AI innovations in biotechnology requires a sophisticated understanding of the technical and legal landscapes and careful strategic consideration of whether patent or trade secret protection better serves business objectives. Patent applications must provide comprehensive technical disclosure while carefully crafting claims that satisfy the distinct requirements of major patent offices. The rapid evolution of both AI technology and patent law necessitates regular review and updating of protection strategies. By focusing on detailed technical implementation, biological validation, and careful claim drafting while strategically choosing what to protect through patents versus trade secrets, companies can build robust intellectual property portfolios that protect their AI innovations while supporting their broader business objectives in the biotechnology space.
Not Legal Advice, No Attorney Client Relationship, for Educational Purposes only.